Humanoid robots are impressive, but they aren’t without challenges in humanoid robotics. One significant challenge is robotic perception, which involves enabling robots to understand and interpret their environment. This article examines the technical, cognitive, and ethical hurdles faced by developers. Expect to learn about achieving stable movement, efficient energy use, human-like interaction, and ethical considerations.
Key Takeaways
- Humanoid robots have historically progressed from simple mechanical designs like Leonardo da Vinci’s 1495 robotic knight to advanced modern robots such as Honda’s ASIMO and Boston Dynamics’ Atlas, showcasing significant technological evolution.
- Key technical challenges in humanoid robotics include achieving stable bipedal locomotion and dynamic balance, ensuring energy efficiency, developing robust control systems, addressing robotic perception, and implementing predictive motor control, all of which are crucial for replicating human-like movements and interactions.
- Cognitive and interaction challenges involve real-time visual recognition, human-robot interaction capabilities, and adaptive learning, which are essential for robots to effectively understand and respond to complex human behaviors and dynamic environments.
Historical Context of Humanoid Robotics
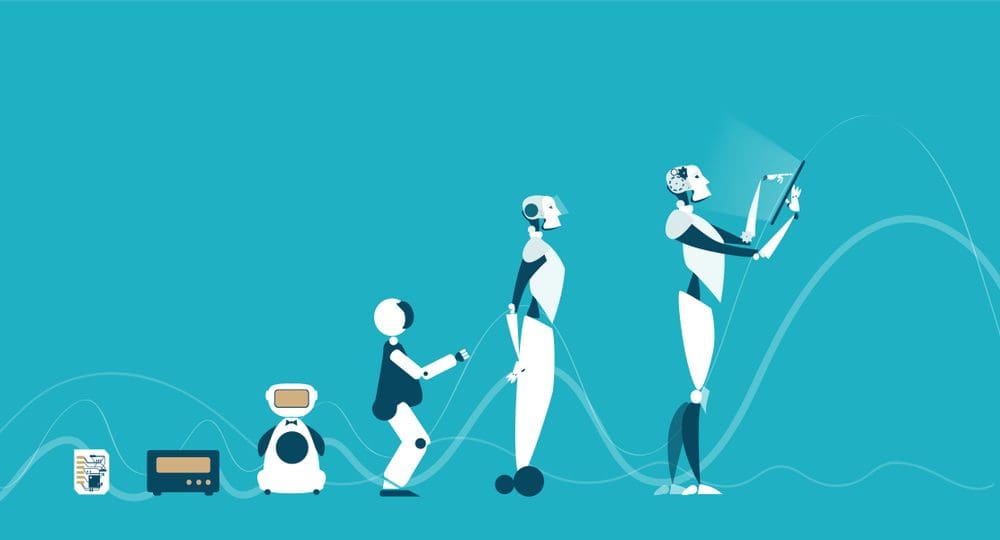
Centuries ago, the earliest recorded designs of humanoid robots emerged, marking the beginning of their journey. Leonardo da Vinci, a visionary far ahead of his time, designed a mechanical knight around 1495 that could perform basic movements. This creation marked the inception of attempts to emulate human-like movements in machines.
In 1810, Friedrich Kaufmann captured many imaginations by introducing a humanoid robot soldier capable of playing the trumpet. The 20th century witnessed significant advancements, with W. H. Richards unveiling one of the first humanoid robots named Eric in 1928. Eric had movable hands and a head controlled by electromagnets, showcasing early attempts at integrating complex mechanical systems into humanoid forms.
Another notable milestone was Elektro, a humanoid robot created for the 1939 World’s Fair. Elektro could walk, speak over 700 words, and perform various tasks via voice command, pushing the boundaries of what was possible at the time. The mid-20th century marked the birth of digital and programmable robots, with George Devol’s invention of Unimate in 1954. Unimate laid the groundwork for modern robotics, revolutionizing the field with its ability to be programmed for different tasks.
As the millennium turned, Honda introduced ASIMO, a humanoid robot with the ability to walk, run, and interact with humans. ASIMO represented a significant leap in humanoid robotics, combining advanced mechanical design with sophisticated control systems to achieve unprecedented levels of human-like movement and interaction. Today, companies like Boston Dynamics and SoftBank Robotics are at the forefront of humanoid robotics, creating machines that not only mimic human gestures and movements but also exhibit a degree of cognitive capability and interaction proficiency.
These advancements are not just technological marvels but also testaments to the relentless pursuit of creating robots that can seamlessly integrate into human environments and augment our capabilities.
Technical Hurdles in Humanoid Robot Development
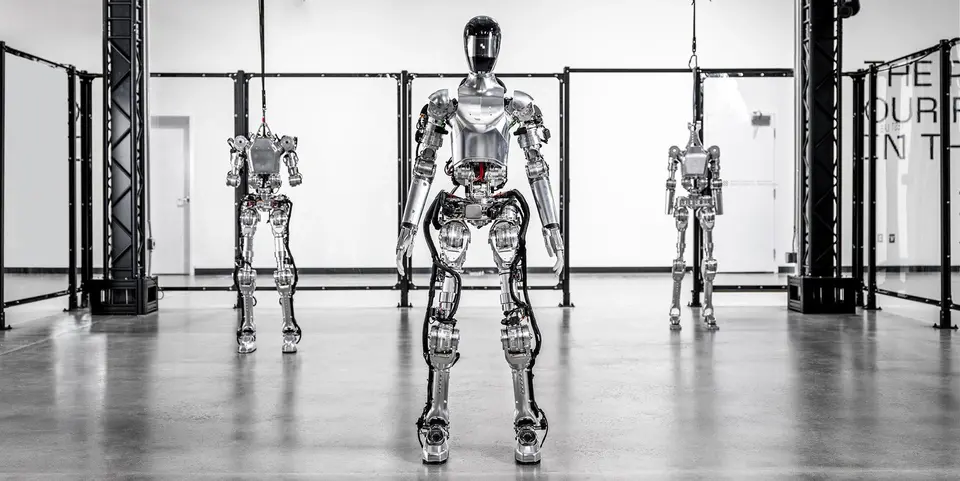
The development of humanoid robots is fraught with technical challenges that require innovative solutions. Some of these challenges include:
- Managing kinematic redundancy and end-effector trajectories to replicate human-like movements
- Achieving dynamic balancing through precise synchronization of actuators, sensors, and control algorithms
- Overcoming actuator saturation and implementing predictive motor control to enable high-intensity tasks and quick maneuvers
These challenges highlight the complexity of creating humanoid robots that can perform tasks with the same level of dexterity and agility as humans in their human form, emphasizing the importance of human-robot coordination.
Effective wear and tear prediction is also essential for maintaining the robots’ longevity and reliability.
Bipedal Locomotion and Stability
Achieving stable bipedal locomotion in humanoid robots is a complex task that involves addressing challenges such as kinematic redundancy, maintaining the Zero Moment Point (ZMP), and Center of Mass (CoM) stabilization. These concepts are crucial for ensuring that the robot does not tip over while walking or standing still. Advanced control algorithms and real-time feedback mechanisms are employed to adjust the robot’s CoM, allowing it to navigate and maintain stability on uneven terrains.
Boston Dynamics has made significant strides in this area with their robots, which are capable of adaptive walking and maintaining balance even on rough terrain. By continuously analyzing feedback from sensors and adjusting their movements accordingly, these robots exhibit a natural gait that closely mimics human-like movements.
Energy Efficiency in Humanoid Robots
For humanoid robots to operate for extended periods of time, energy efficiency is a crucial factor. The performance and control of actuators play a significant role in determining how efficiently a robot uses its energy. Minimizing actuator saturation and ensuring efficient motion control are essential for optimizing energy consumption.
Robots like Boston Dynamics’ Atlas have demonstrated remarkable energy efficiency, allowing them to perform complex tasks without depleting their power reserves quickly. This is achieved through the use of advanced materials and control systems that minimize energy waste and maximize output.
Robust Control Systems
Having robust control systems in humanoid robots is of paramount importance. These systems manage the intricate interplay between kinematics, sensors, and actuators, ensuring that the robot can perform tasks with precision and reliability. Integrating advanced sensory systems for comprehensive environmental perception is a complex task that requires sophisticated algorithms and sensor fusion techniques.
Sensors such as inertial measuring units (IMUs), LiDAR, and cameras are used to provide a thorough picture of the robot’s environment, enabling it to move about, communicate, and make decisions. Translating human dexterity and manipulation capabilities into robotic systems also requires intricate mechanical design and control strategies.
Cognitive and Interaction Challenges
Humanoid robots face significant cognitive and interaction challenges, particularly in understanding and processing complex human behaviors, interactions, and contextual awareness in real-time. Equipping robots with contextual awareness to understand human emotions and decision-making processes is a formidable task that involves developing advanced cognitive capabilities and interaction proficiencies.
Real-Time Visual Recognition and Processing
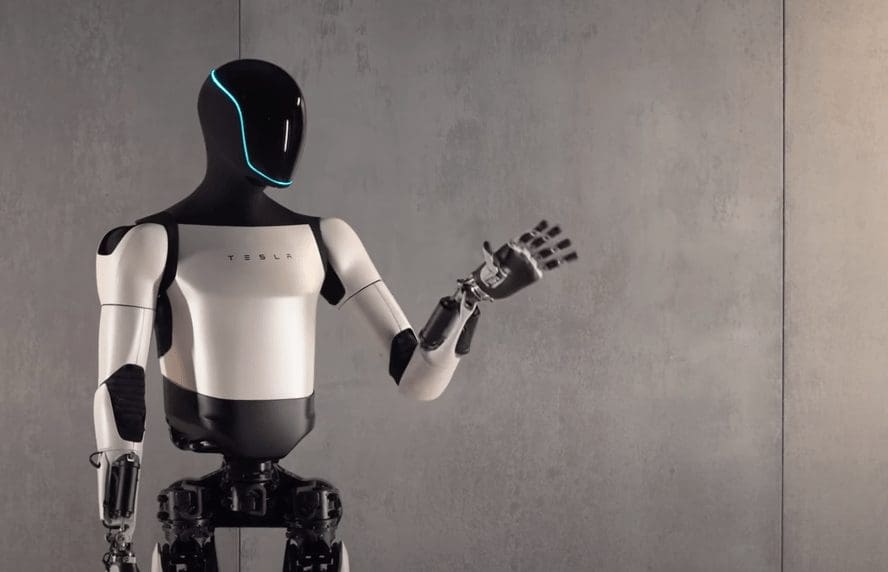
For humanoid robots to interact effectively with their environment, they need to have real-time visual recognition and processing capabilities. Building invariant models of observed scenes, often utilizing 3D information, enhances accuracy and allows robots to recognize and interpret human gestures accurately. Techniques such as sparse coding and pooling are employed to maintain low computational costs while performing these tasks in real-time.
Combining RGB and depth information (RGB+D) significantly improves visual recognition accuracy, although it imposes higher computational demands. Sophisticated algorithms are essential for identifying and interpreting human gestures, enabling robots to react promptly to human actions and requests.
Human-Robot Interaction Capabilities
Humanoid robots designed to collaborate with humans require effective human-robot interaction (HRI). These robots must excel in understanding human gestures, expressions, and intentions to interact seamlessly with human partners. Multimodal communication, which includes visual, vocal, and physical channels, plays a vital role in facilitating effective cooperation.
Robots like Ameca and Pepper have set benchmarks in HRI. Ameca, a product of Engineered Arts, uses generative artificial intelligence to respond to queries and carry out imaginative tasks, showcasing advanced interaction capabilities. Pepper, from Softbank Robotics, is used in educational and medical environments to identify faces, monitor emotions, and provide contactless care and communication.
Imitation learning is another technique that enhances HRI by allowing robots to acquire new skills through observation and mimicry. This adaptive capability is crucial for robots intended to work alongside humans in dynamic environments.
Adaptive Behavior and Learning
For humanoid robots to navigate and respond to dynamic environments, adaptive behavior and continuous learning are vital. Machine learning techniques, such as deep reinforcement learning (DRL), enable robots to learn adaptive strategies in uncertain and complex settings. This robot machine learning capability allows robots to make decisions and perform tasks autonomously, enhancing their utility in real-world applications.
Developing adaptive behavior requires methods that can be learned from a small set of examples without extensive training. This approach ensures that robots can quickly adapt to new situations and environments, making them more versatile and effective in various roles.
Ethical and Social Considerations
The deployment of humanoid robots comes with significant ethical and social considerations. Ensuring that these robots act in the best interest of society requires clear ethical guidelines and accountability measures.
The emotional connection between humans and robots raises questions about the emotional well-being of both users and robots, highlighting the need for thoughtful ethical considerations and taking into account human psychological aspects.
Ethical Domains and AI Transparency
To build user trust and ensure ethical use of humanoid robots, AI algorithms must be transparent. Transparent algorithms help users understand the decision-making processes of robots, fostering trust and accountability. Safeguarding privacy is also vital, as robots often process and store vast amounts of personal data.
Developers must ensure that humanoid robots align with ethical guidelines to maintain accountability and protect user privacy. This involves balancing data-driven insights with the protection of personal information, ensuring that robots act responsibly and ethically.
Impact on Employment and Society
The rise of humanoid robots poses significant economic impacts, particularly on job markets. While these robots can perform repetitive tasks and enhance productivity, they also have the potential to displace jobs, especially in roles involving routine tasks. This necessitates ethical discussions and proactive measures by policymakers to mitigate negative consequences.
Policymakers must address the potential for economic inequality and job displacement caused by the widespread use of humanoid robots. At the same time, new job opportunities in the robotics industry, such as roles in robot design, programming, and maintenance, can emerge, offering avenues for economic growth and employment.
Advanced Research and Technological Innovations
Recent advancements in humanoid robotics have pushed the boundaries of what these machines can achieve. From biomimetic design and mechanical engineering to neural networks and multi-robot cooperation, the field is witnessing rapid progress. These innovations are not only enhancing the capabilities of humanoid robots but also expanding their potential applications in various industries.
Multi-agent reinforcement learning is playing a crucial role in advancing humanoid robotics by enabling more sophisticated and coordinated behaviors among multiple robots.
Biomimetic Design and Mechanical Engineering
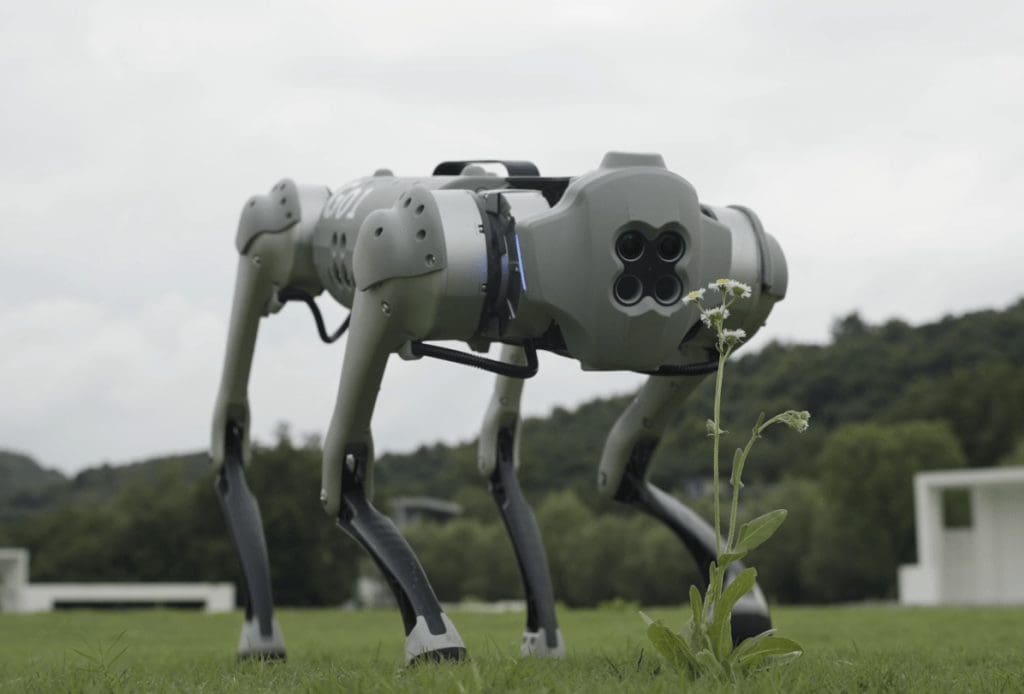
In robotics, biomimetic design is used to mimic biological systems, thereby creating more adaptable and efficient robotic mechanisms. This approach is evident in robots like Ameca, which closely mimic human facial expressions and movements using advanced materials and sophisticated mechanical engineering.
Achieving natural human-like motion in humanoid robots demands advancements in motor control, kinematics, and understanding of human movement. Robots like ALTER 3 and ARMAR-6 use sophisticated sensors, strong computing units, and precise actuators to enhance their mechanical performance and interaction capabilities.
Neural Networks and Autonomous Control
To develop adaptive behavior and autonomous control in humanoid robots, machine learning and neural networks are indispensable. Deep learning models, such as convolutional neural networks (CNN), have significantly improved robots’ perception, decision-making, and control capabilities. Neural networks enable robots to learn complex decision-making strategies from data, enhancing their autonomous control.
1X’s humanoid robots utilize vision-based neural networks for real-time autonomous control of movements. These robots learn motor behaviors directly from visual input through end-to-end learning from data, showcasing the potential of neural networks in advancing humanoid robotics.
Multi-Robot Cooperation and Coordination
For task efficiency improvement and achieving complex objectives unmanageable by a single robot, multi-robot cooperation and coordination are vital. Some benefits of multi-agent reinforcement learning (MARL) models include:
- Enhancing collaborative decision-making and interaction among multiple robots
- Enabling robots to work together seamlessly
- Facilitating synchronized actions and shared resources
This collaborative approach is essential for tasks that require synchronized actions and shared resources.
Distributed control systems further enhance multi-robot cooperation by decomposing tasks into subtasks and coordinating their execution. This ensures that multiple robots can operate efficiently, handle complex tasks, and adapt to dynamic environments, thereby significantly improving overall task efficiency and effectiveness.
Real-World Applications and Future Prospects
The real-world applications of humanoid robots are vast and varied, ranging from industrial automation and collaborative robots to healthcare and education. These robots can augment human capabilities, address labor shortages, and perform tasks in environments that are hazardous or difficult for humans.
As technology continues to advance, the future prospects for humanoid robots are incredibly promising, with potential applications expanding into new and innovative areas.
Industrial Automation and Assistance
Humanoid robots, also referred to as collaborative robots or cobots, are designed to work alongside humans in industrial settings, boosting productivity without necessarily replacing jobs. These robots can assist with repetitive and laborious tasks in manufacturing and logistics, such as assembly line work, sorting, and transporting items. By mimicking human motion and interaction, humanoid robots can perform these tasks with precision and efficiency, leading to significant cost savings and productivity gains.
Additionally, humanoid robots play a crucial role in reducing workplace injuries by taking over tasks that involve heavy lifting or repetitive motions. In warehouses, they improve efficiency and accuracy by sorting and transporting items, thereby streamlining operations and reducing the risk of human error.
Healthcare and Elderly Care
Humanoid robots can assist healthcare professionals by:
- Lifting and transporting patients, thereby reducing the risk of injuries and enhancing patient care.
- Assisting with lifting and transferring patients in elderly care.
- Providing emotional support and companionship to the elderly.
- Alleviating feelings of loneliness and improving overall well-being.
Therapeutic robots like Paro are used specifically to provide emotional support and companionship to the elderly.
The integration of humanoid robots in healthcare not only enhances the quality of care but also reduces the physical strain on healthcare workers, allowing them to focus on more critical tasks. As these robots continue to evolve, their role in healthcare and elderly care is expected to expand, offering new possibilities for patient support and care delivery.
Education and Public Service
Humanoid robots can enrich educational settings by providing interactive lessons and assisting students with special needs. These robots can engage students with diverse learning styles through interactive and personalized teaching methods, enhancing the educational experience. In public service roles, humanoid robots are increasingly being used in libraries and museums to guide visitors and provide information, making these environments more accessible and engaging.
The potential of humanoid robots in education and public service is vast, offering opportunities to enhance learning experiences and improve public interactions. As technology advances, these robots will likely become more integrated into educational institutions and public spaces, providing valuable support and services to people of all ages.
Summary
The development and deployment of humanoid robots presents both exciting opportunities and significant challenges. From their historical evolution to the technical, cognitive, and ethical hurdles they face, humanoid robots are a testament to human ingenuity and the relentless pursuit of innovation. As we continue to refine their capabilities and address the associated ethical and social considerations, these robots hold the potential to transform various industries, enhance human capabilities, and improve quality of life.
The future of humanoid robotics is bright, with ongoing research and technological advancements paving the way for even more sophisticated and versatile robots. By embracing these advancements and addressing the challenges head-on, we can unlock the full potential of humanoid robots, creating a future where humans and robots work together in harmony to achieve common goals.
Frequently Asked Questions
What are some historical milestones in the development of humanoid robots?
Some historical milestones in the development of humanoid robots include Leonardo da Vinci’s mechanical knight from 1495, Friedrich Kaufmann’s humanoid robot soldier from 1810, W. H. Richards’ Eric from 1928, and Honda’s ASIMO from 2000.
What are the main technical challenges in developing humanoid robots?
The main technical challenges in developing humanoid robots include replicating human-like movements, achieving dynamic balancing, optimizing energy efficiency, and developing robust control systems. This involves addressing complex engineering requirements.
How do humanoid robots achieve real-time visual recognition?
Humanoid robots achieve real-time visual recognition by building invariant models using 3D information, sparse coding, and pooling techniques to maintain low computational costs and enhance accuracy. To do this, process visual data quickly while maintaining high precision.
What ethical considerations are associated with humanoid robots?
When considering humanoid robots, it’s important to address transparency in AI algorithms, privacy protection, emotional well-being, and the impact on employment and economic inequality. These are key ethical considerations.
What are some real-world applications of humanoid robots?
Real-world applications of humanoid robots include industrial automation, healthcare, elderly care, education, and public service roles. They assist with tasks such as patient care, interactive lessons, and guiding visitors in public spaces.